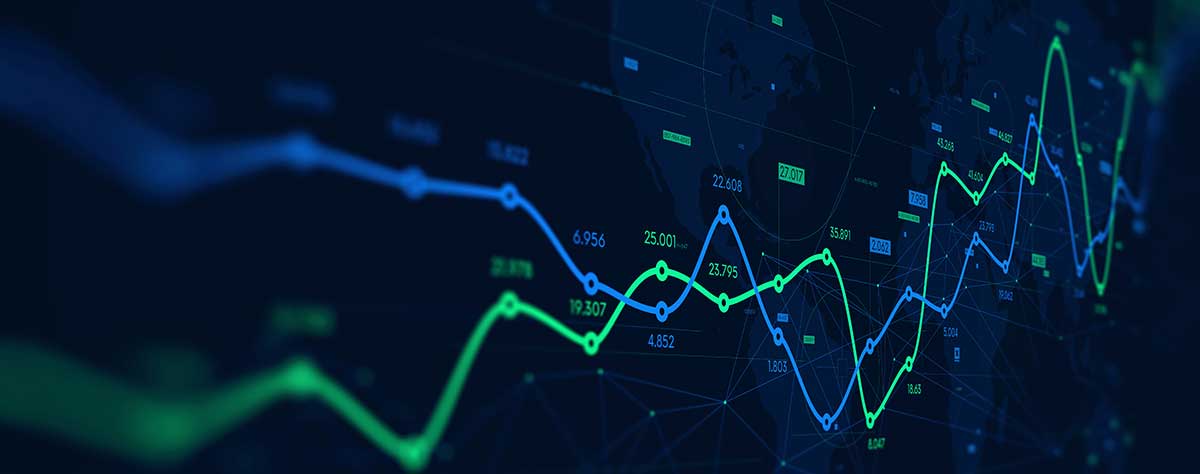
Events
Dr. Wenbo Wu, University of Texas at San Antonio presents On Partial Envelope Approach for Modeling Spatial-Temporally Dependent Data
Date: Thursday, February 22, 2024
Dr. Anton Westveld, Australian National University presents Bayesian Melding: Deterministic to the Stochastic Case
Date: Thursday, November 9, 2023
Dr. Hongxiao Zhu, Associate Professor, Virginia Tech presents Region Detection on Functional Data
Date: Thursday, November 2, 2023
Dr. Shan Yu, Department of Statistics, University of Virginia presents Big Spatial Data Learning: A Parallel Solution
Date: Thursday, September 21, 2023
Check out our past events.